Words by David Hughes
Avionics systems that use artificial intelligence are being developed for up-and-coming eVTOL aircraft, but general aviation piston aircraft will be the first users.
President and founder of avionics company Avidyne Dan Schwinn heard about Daedalean’s work in artificial intelligence (AI) avionics in 2016. He traveled from Avidyne’s base in Florida, USA to visit the Swiss company in Zurich in 2018. The two companies formed a partnership to develop the PilotEye system in 2020.
PilotEye is a computer vision-based system that detects, tracks and classifies fixed-wing aircraft, helicopters and drones. Avidyne is aiming to gain FAA certification for the system this year with concurrent validation by EASA.
“The target is still certification this year, but due to the novelty of the system there is some risk to that,” said Schwinn. “We expect to have finalized systems by the middle of the year. Development, validation and certification activities are very active in the STC [Supplemental Type Certificate] program underway at FAA and EASA.”
Visual acquisition
Schwinn founded Avidyne 27 years ago to bring big glass cockpit displays to general aviation (GA) cockpits, initially on the Cirrus SR20 and SR22. Avidyne has decades of experience certifying GA avionics and producing and servicing systems in the field.
PilotEye functions will integrate with any standards-based traffic display. It can be installed on a traditional flight deck to visually acquire traffic using cameras and AI computer vision while allowing the pilot to use an iPad to zoom in on traffic. There will be some enhanced features available when it is installed with Avidyne displays.
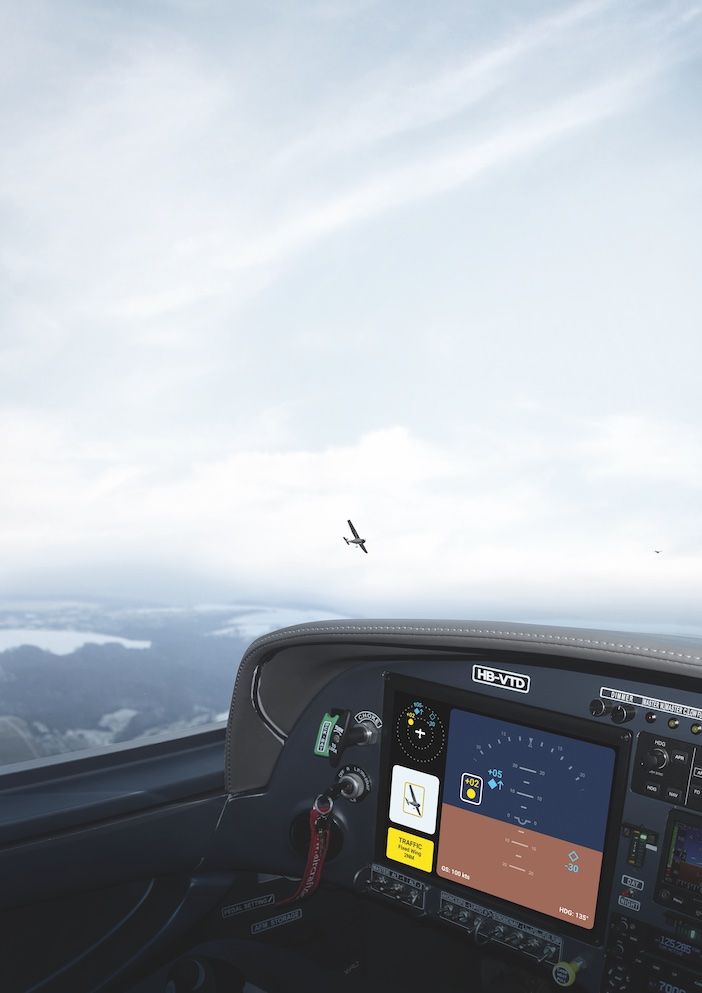
PilotEye can detect a Cessna 172 at 2 miles (3.2km) and a Group 1 drone (20 lbs, 9kg) at a few hundred yards. The system will eventually be coupled to an autopilot to facilitate collision avoidance in an aircraft. PilotEye can also detect some types of obstacles.
For PilotEye’s flight test programs Avidyne installs the traditional avionics hardware, while Daedalean provides the neural network software.
“There have been neural networks for analyzing engine data but not for a real time, critical application like PilotEye,” Schwinn said.
“I believe this will be the first of its type. We have put a lot of effort into this and we know how to do the basic blocking and tackling of aircraft installation and certification.”
Once the system is certified with visual cameras as the sensors, Avidyne may add infrared or radar sensors as options. Avidyne has flown hundreds of hours of flight tests with PilotEye and done thousands of hours
of simulation.
The company is getting a lot of interest in the system from helicopter operators, who fly at low altitude and often encounter non-cooperative targets. PilotEye’s forward-facing camera has a 60˚ field of view and the two side-facing cameras have 80˚ fields of view, which produces a 220˚ panorama. The system will have three cameras initially and an optional fourth camera later which helicopter operators might want to point down to find helipads or potential emergency landing locations.
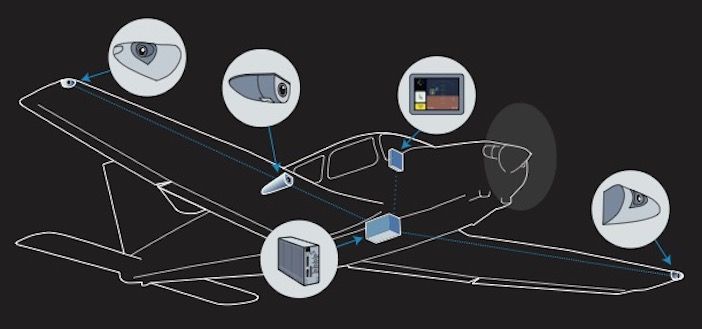
Neural networks
Startup Daedalean has been developing neural network technology for aviation since 2016, mainly as flight control systems for autonomous eVTOL aircraft. The company’s increasingly automated flight control systems are driven by AI and machine learning.
Engineers at Daedalean have conducted extensive simulation and flight testing of its own visual AI software and hardware. It provides an evaluation kit of its computer vision based situational awareness system, along with drawings and documentation so airframe, avionics companies and large fleet operators and holders of STCs and Type Certificates can install it on their own flight test aircraft. Last year Embraer and its UAM subsidiary Eve logged seven days of flight tests in Rio de Janeiro with Daedalean and other partners to evaluate autonomous flight in an urban environment.
The two-camera evaluation kit provides visual positioning and navigation, traffic detection and visual landing guidance portrayed on a tablet computer in real time. Installation is not easy and involves more than duct tape to ensure it is safe for flight. The kit can also be integrated with flight control instruments at any level desired.
Daedalean can help with custom mountings, enclosures and support on request. End users can buy or rent the evaluation kit or partner with Daedalean long-term in developing advanced situational awareness systems.
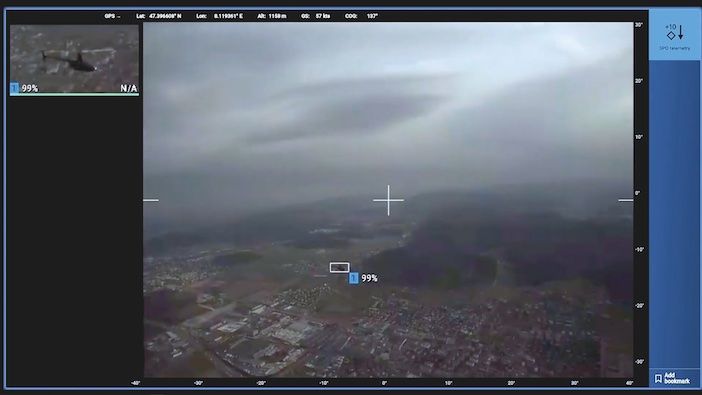
Data everywhere
Daedalean knows it needs end users involved in the process to perfect the technology. An aim for the firm is to leverage end-user flight data to help assess the performance of the computer vision technology in real world scenarios.
The developmental system Daedalean has been testing has one to four cameras and a computing box which weigh around 15 lbs (6.5kg). The equipment is a Level 1 AI/Machine learning system. As defined by EASA, Level 1 provides human assistance. Level 2 is for human/machine collaboration and Level 3 is a machine able to perform decisions and actions fully independently.
Avidyne’s joint project is classed as Level 1. Daedalean doesn’t expect a Level 3 system for eVTOL aircraft to be ready for certification until 2028.
eVTOL aircraft developers have several novel areas within aircraft designs that needed development and testing as well as machine-learning avionics – new designs, flight controls, noise, propulsion systems – to name but a few. This is why Avidyne’s Level 1 autonomous PilotEye system will be seen first on traditional general aviation aircraft.
Daedalean has collected around 500 hours of flight test video recordings in rented GA aircraft and helicopters for its situational awareness system. From this it has gathered 1.2 million still images taken during 7,000 encounters between the data gathering aircraft and another aircraft providing
the target.
The data recording equipment gathers six images per second during the 10-20 second encounters with varied altitudes, directions and speeds. These images are examined post-flight by human analyzers who identify the aircraft in the image. After this a neural network statistical analyzer reviews each pixel and relates them to one another to answer the question, “is there an aircraft in the image?” An algorithm, unlike a human eyeballing a target, can analyze millions of parameters and can provide reliability similar to human observation.
The code is then frozen and released to partners using Daedalean evaluation kits. Feedback from these users guides the next release. Updates happen several times a year.
As development work continues, the aim is to eventually have the system interfaced with flight controls to avoid hazards such as obstacles and terrain. The pilot’s role will become limited and then there will be no pilot aboard the aircraft. The system will also communicate with air traffic control and to other Daedalean equipped aircraft.
Certification routes
Daedalean is working with regulators, including EASA’s AI task force to develop an engineering process
to be applied to AI and machine learning avionics during certification.
The standard software development process follows a V-shaped method which confirms software conforms to requirements, standards and procedures. It applies to the life cycle of software, from writing code through verification and validation.
AI and machine learning avionics software raises new certification challenges. EASA and Daedalean have created a W-shaped process to guide certification efforts. The middle of the W is used to verify the learning process and ensure that the learning technique is correctly applied.
The AI application needs to work correctly in more than 99% of cases with the precise figure determined by the safety critical level of a particular function. See EASA AI Task Force/Daedalean reports titled “Concepts of Design Assurance for Neural Networks (CoDANN).” CoDANN Report 1 in 2020, Report 11 published in 2021.
The FAA also worked with Daedalean in 2022 on research that assessed the W-shaped learning assurance process for future certification policy. The FAA has also evaluated whether visual-based AI landing assistance would backup other navigation systems during a GPS outage. The team flew 18 computer vision landings during two flights in an Avidyne flight test aircraft in Florida. The resulting report “Neural Network Based Runway Landing Guidance for General Aviation Autoland” is available on the FAA website.
Avionics supplier Honeywell has also partnered with Daedalean to develop and test avionics for autonomous takeoff and landing, as well as GPS-independent navigation and collision avoidance.
Honeywell Ventures is an investor in Daedalean. Last year the Swiss company established a US office near Honeywell’s headquarters in Phoenix, USA.
The FAA is also involved in working to bring AI and neural network machine learning to general aviation cockpits by funding R&D with US research agency MITRE.
Software engineer Matt Pollack has been working on the digital copilot project that began in 2015. According to Pollack the work aims to aid pilots in the form of a portable device. Half of the MITRE team are software engineers and half are human factors specialists. There are also GA pilots in the team,
and Pollack is an active commercial multi-engine pilot and a CFII.
Flight testing of the first algorithms began in 2017 with a Cessna 172 and since then a total of 50 flight test hours have been conducted in light aircraft as well as in helicopters.
The cognitive assistance provided by the digital co-pilot operates like Apple’s Siri or Amazon’s Alexa voice assistance do on the ground. It aids a pilot’s cognition without replacing it and is enabled by automatic speech recognition and location awareness.
A lot of existing data is fed into the device including the flight plan, NOTAMS, PIREPS weather, traffic data, geolocation, and high accuracy GPS, AHRS and ADS-B data including TIS-B, FIS-B. The MITRE developed algorithms include speech recognition technology and the context of what information a pilot needs during a particular phase of flight. The algorithms provide useful information with audio and visual notifications based on the context of what the pilot is trying to accomplish.
The information is not prescriptive in telling the pilot what to do. For example, weather information may indicate there are deteriorating conditions along the route of flight such as reduced visibility or cloud cover.
It may be a good time for the pilot to come up with an alternative routing but the digital copilot won’t tell him what to do.
The system can also provide a memory prompt. If a controller tells a pilot to report 3 miles (4.8km) on a left base, the digital copilot can listen to that radio call and look up the reporting point on a map. It then provides a visual or aural reminder when the aircraft approaches that point.
The MITRE team has created 60 different features in algorithms so far and has been in conversations with companies that provide mobile avionics devices, as well as some who provide panel mounted avionics. Foreflight has already adopted some of the MITRE features into its products. Companies can obtain the technology through the MITRE’s technology transfer process for use under license.
The aim of the features developed are to reduce workload, task time or increase awareness and heads-up time. There are three types of assistance cues: on-demand information, contextual notifications and hybrid reminders that combines the characteristics of the first two.
In 2022, Pollack wrote an FAA technical paper “Cognitive Assistance for Recreational Pilots,” with two of his MITRE colleagues Steven Estes and John Helleberg. They wrote that: “Each of these types of cognitive assistance are intended to benefit the pilot in some way – for example by reducing workload, reducing task time or increasing awareness and head-up time”.
MITRE expects design standards to evolve as AI advances. It has been testing neural networks and machine learning algorithms for use in aviation and sees a whole host of issue to be addressed there.