Researchers from the European Space Agency are racing drones at Delft University of Technology in the Netherlands to test the performance of AI control systems for space missions.
The European Space Agency’s (ESA’s) Advanced Concepts Team is working with the Micro Air Vehicle Laboratory (MAVLab) at TU Delft and using its Cyber Zoo to test the drones and tweak the AI algorithms.
Dario Izzo, scientific coordinator of ESA’s ACT said, “We have been looking into the use of trainable neural networks for the autonomous oversight of all kinds of demanding spacecraft maneuvers, such as interplanetary transfers, surface landings and dockings.
“In space, every onboard resource must be used as efficiently as possible – including propellant, available energy, computing resources and often time. Such a neural network approach could enable optimal onboard operations, boosting mission autonomy and robustness. But we needed a way to test it in the real world, ahead of planning actual space missions.
“That’s when we settled on drone racing as the ideal gym environment to test end-to-end neural architectures on real robotic platforms, to increase confidence in their future use in space.
Drones have been competing to achieve the best time through a set course within the Cyber Zoo, a 10x10m (33 x 33ft) test area. Human-steered quadcopters were alternated with autonomous counterparts with neural networks trained in various ways.
“The traditional way that spacecraft maneuvers work is that they are planned in detail on the ground then uploaded to the spacecraft to be carried out,” said ACT young graduate trainee Sebastien Origer. “Essentially, when it comes to mission Guidance and Control, the Guidance part occurs on the ground, while the Control part is undertaken by the spacecraft.”
Space is inherently unpredictable, with the potential for all kinds of unforeseen factors and noise, such as gravitational variations, atmospheric turbulence or planetary bodies that turn out to be shaped differently from on-ground modeling.
Whenever the spacecraft deviates from its planned path for whatever reason, its control system works to return it to the set profile. The challenge is that this can be quite costly in resource terms and requires brute-force corrections.
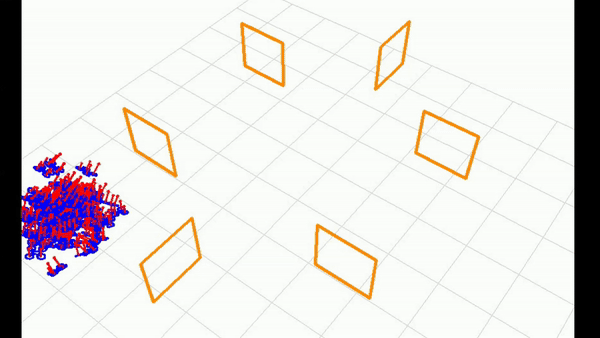
“Our alternative end-to-end Guidance & Control Networks [G&CNET] approach involves all the work taking place on the spacecraft. Instead of sticking a single set course, the spacecraft continuously replans its optimal trajectory, starting from the current position it finds itself at, which proves to be much more efficient,” said Origer.
In computer simulations neural nets composed of interlinked neurons – mimicking the setup of animal brains – performed well when trained using ‘behavioral cloning’, based on prolonged exposure to expert examples.
Validation of the simulations required the use of the drones. “There’s quite a lot of synergies between drones and spacecraft, although the dynamics involved in flying drones are much faster and noisier,” said Izzo.
“When it comes to racing the main scarce resource is time, but we can use that as a substitute for other variables that a space mission might have to prioritize, such as propellant mass.
“Satellite CPUs are quite constrained, but our G&CNETs are surprisingly modest, perhaps storing up to 30 000 parameters in memory, which can be done using only a few hundred kilobytes, involving less than 360 neurons in all.”
The G&CNet sends commands directly to the actuators – the equivalent for a spacecraft are the thrusters and their propellers in the case of drones.
“The main challenge that we tackled for bringing G&CNets to drones is the reality gap between the actuators in simulation and in reality”, said Christophe De Wagter, principal investigator at TU Delft.
“We deal with this by identifying the reality gap while flying and teaching the neural network to deal with it. For example, if the propellers give less thrust than expected, the drone can notice this via its accelerometers. The neural network will then regenerate the commands to follow the new optimal path.”